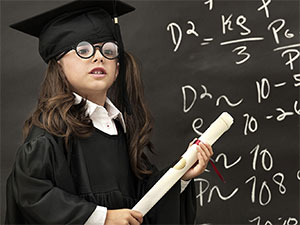
ChessBase 17 - Mega package - Edition 2024
It is the program of choice for anyone who loves the game and wants to know more about it. Start your personal success story with ChessBase and enjoy the game even more.
An interesting phenomenon of the Elo rating system is that when examining game outcomes for a group of juniors, they typically overperform when they are lower rated. Using statistical methods based on game results for junior-junior, junior-adult and adult-adult pairings, I find that on average juniors are statistically underrated by up to 50 Elo rating points against adult players, and this bias increases with the age difference. Rating systems that have potential in solving the bias and noise elements are a momentum based system or a Glicko system.
Continuing on from my findings in my previous article(1), I have expanded my dataset to include the age of both players at the time their game is played using Chessbase’s Megabase 2016(2). This is useful as by identifying the opponents’ age I can now examine the game results of 3 groups, junior-junior, junior-adult and adult-adult pairings(3). I hypothesize that junior-adult pairings should show a systematic bias in that lower rated juniors are overperforming relative to the theoretical Elo formula. This is intuitive as junior players are often making large strides in their development and consequently their current rating lags their true rating by a significant margin. I compute the conditional expectation function (CEF) for each pairing group, which is the expected score conditional on different levels of the rating difference between the two players, and is from the perspective of the junior player for junior-adult pairings (Figure 1). Lower rated juniors perform significantly better than the Elo formula suggests, and this result is more acute for junior-adult pairings. In contrast, adult-adult pairings more closely correspond to the Elo prediction.
Figure 1: CEF for junior-junior, junior-adult and adult-adult pairings
To substantiate the age bias, I can filter my sample of junior-adult pairings into sub-samples of pairings with age differences greater than 10 and 20 years of age. The age bias increases with the age gap in the data (Figure 2), where the probability the junior wins increases by more with a larger age gap. Older players are typically past their peak and so any systematic overrated bias of older players will substatinate this effect.
There is a simple way to incorporate the empirical results to recalibrate the rating formula(4). To start off we need to distinguish between the noise aspect of the results and the systematic undervaluation or bias. The theoretical Elo formula is calibrated so that σ is standardized to 200 rating points for all players(5). This assumption is clearly false in light of the data. One way to make the slope of the Elo prediction flatter is to increase rating variance. In contrast, a bias term will shift the Elo prediction up. I can then calibrate the rating variance and bias parameters to match the CEF for the three groups of pairings.
Figure 2: CEF for Junior-adult pairings with different age gaps
By Elo’s assumption, a player’s rating is normally distributed with mean μ and standard deviation σ. Assuming juniors and adults are distinct groups, the junior’s rating is normally distributed , and the adult’s rating follows the distribution,
. Conceptually, the bias and noise terms can be captured by imposing a higher variance parameter
and a bias
for the junior player. The expected score a junior scores against an adult is given by the following formula, where Φ is the cumulative distribution function of the normal distribution.
I have devised a two-step procedure to estimate the variance and bias parameters for juniors.
The results of this exercise are =276,
=238 and
=48. This suggests that on average the true rating of juniors are 48 Elo rating points above their reported rating. The 95% confidence intervals(6) are tightly clustered around the point estimates, and is [275,277] for
, [237,239] for
and [48,50] for the bias parameter. Plotting the recalibrated Elo formula matches the junior-adult, junior-junior and adult-adult pairings much more closely, as seen in Figure 3.
Figure 3: Recalibrated Elo formula matches CEF for junior-adult and junior-junior pairings
A simplifying assumption of the estimation is treating juniors and adults as two distinct, homogenous groups, when in reality there is a lot of heterogeneity in and
. For example, if we select the subsample of adult-junior pairings with age differences greater than 20, the bias increases to 85 rating points, and when looking at an age difference greater than 30 years of age, the bias increases to 105 points. Now that we know there is a systematic bias in junior ratings, what are some potential solutions?
There are examples of current rating systems that can deal with these rating discrepancies. To address bias, a momentum rating system which weights recent games of a player would be effective. For example, if there is a statistically significant difference between a player’s performance rating and their current rating after a threshold number of games, the expected score can be modified to take into account this performance rating instead of the player’s actual rating. An alternative rating system is the Glicko rating system devised by statistician Mark Glickman(7) and is currently used by the Australian Chess Federation. Using games played during a rating period his algorithm estimates a player volatility parameter that attempts to estimate each player’s individual σ to then calculate the expected score for rating calculations. The system was originally intended to address the issue of a player coming back to chess after a number of years, with their player variance increasing over time, however it can be generalized to situations where players can be underrated due to rapid improvement. Another advantage of this system is that rating changes do not have to be symmetric, as the K factor is now proportional to player variance. These solutions are by no means exhaustive and I believe there is more work to be done in terms of optimizing rating systems to address the empirical concerns in this article.
(1) See http://en.chessbase.com/post/Elo-rating-system-how-underrated-are-the-kids
(2) The only way I could do this was to create a database of players born in each year and record their set of game results. I then merge databases for each year to then identify the opponent’s age. The sample size for each group are 574,094 games for junior-adult pairings, 358,445 games for junior-junior pairings, and 317,113 games for adult-adult pairings.
(3) Juniors are defined under 18 years of age. I have only included subsample of players born in 1960 onwards in my analysis.
(4) I am aware there are numerous methods to use the data in Figures 1 and 2 to statistically estimate the age effect. For example, a different way to estimate the age bias is by logistic regressions with an age dummy as well as interacting age with the rating difference. My main concern is that it is hard to isolate the age bias from the noise in the data, and the rating formula you derive from a logistic regression deviates significantly from a more standard Elo formula.
(5) The Elo formula uses a logistic function and so slightly deviates from the Normal cumulative distribution function at high rating differences.
(6) Intervals constructed using non-parametric bootstrap with 1000 simulations.
(7) For more details, see http://www.glicko.net/glicko/glicko2.pdf
![]() |
About the author Ganesh Viswanath is from Perth, Australia and is currently studying a PhD in economics from the University of California, Berkeley. In his spare time he likes to play chess tournaments in the bay area (facing a lot of juniors!) and has more recently been trying to venture into chess statistics. |