Chess Festival Prague 2025 with analyses by Aravindh, Giri, Gurel, Navara and others. ‘Special’: 27 highly entertaining miniatures. Opening videos by Werle, King and Ris. 10 opening articles with new repertoire ideas and much more.
ChessBase Magazine offers first-class training material for club players and professionals! World-class players analyse their brilliant games and explain the ideas behind the moves. Opening specialists present the latest trends in opening theory and exciting ideas for your repertoire. Master trainers in tactics, strategy and endgames show you the tricks and techniques you need to be a successful tournament player! Available as a direct download (incl. booklet as pdf file) or booklet with download key by post.
Included in delivery: ChessBase Magazine #225 as “ChessBase Book” for iPad, tablet, Mac etc.!
ChessBase Magazine offers first-class training material for club players and professionals! World-class players analyse their brilliant games and explain the ideas behind the moves. Opening specialists present the latest trends in opening theory and exciting ideas for your repertoire. Master trainers in tactics, strategy and endgames show you the tricks and techniques you need to be a successful tournament player! Available as a direct download (incl. booklet as pdf file) or booklet with download key by post.
Included in delivery: ChessBase Magazine #225 as “ChessBase Book” for iPad, tablet, Mac etc.!
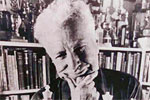