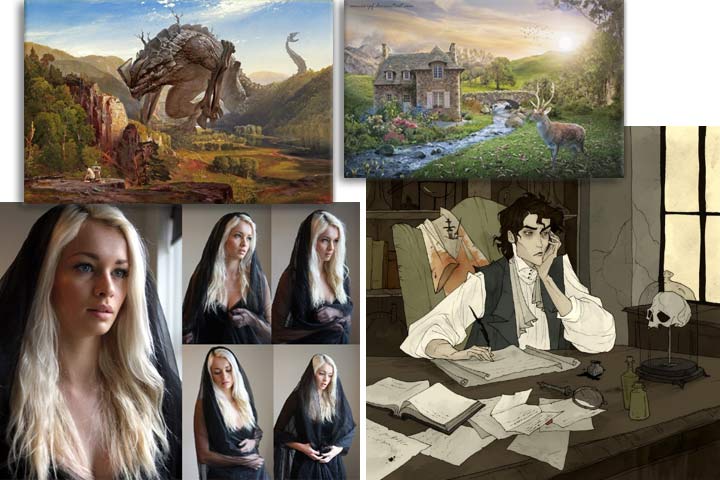
Winning starts with what you know
The new version 18 offers completely new possibilities for chess training and analysis: playing style analysis, search for strategic themes, access to 6 billion Lichess games, player preparation by matching Lichess games, download Chess.com games with built-in API, built-in cloud engine and much more.
Recently, I decided to try Chesthetica’s ‘study’ composing feature using only the pieces from the study by John Roycroft from July 1965, which was the first published by EG magazine and was the topic of ChessBase's December 2017 Study of the Month column. The only difference from the last time I used Chesthetica for something like this is that I increased the number of Deviant Art images used since then to 340 (from 300) because I found a few more that I happened to like for their creative ‘material’ if you will.
The question in my mind was whether Chesthetica could come up with original study-like constructs (using the same pieces from an actual published study) that not only demonstrated some level of creativity in chess but was also challenging to players, in general. Not exclusively to master composers, mind you, as they represent only a very small subset of the chess-playing global community. It is also noteworthy that by ‘creativity’, I mean the kind of compositions that would impress us if say, they had been produced independently by a 12-year-old child who had been playing for a few years. It should be pointed out that Chesthetica, even though a computer program and not a child, probably only ever had less than 0.1% of Deep Blue or Alpha Zero’s total resources behind it. Today, it’s basically just me and a couple of personal computers I have access to.
As I’ve explained before, but feel compelled to repeat here again, Chesthetica does not use any specialized ‘composing knowledge’ related to studies other than optionally filtering out those that don’t abide by some common conventions (e.g. no ‘check’ in the key move) and of course, being able to determine if a composed position is legal or not. There was no ‘training data’ either. While it can evaluate aesthetics in move sequences to some extent (Chesthetica began development in 2006 or so as just an aesthetics evaluator in the game), it does not use any of its aesthetic or thematic game principles in the composing process.
Consider how an art critic might be able to evaluate a Monet or Picasso but probably would not be able to use that knowledge to paint one himself. Put simply, Chesthetica uses the DSNS and the ‘creative attribute fragments’ from the constituent domains employed (in this case the usual 1,000 tournament games sequences between weak players and 340 Deviant Art photos) to help decide where to place the pieces when trying to compose a problem. This is analogous to a human who may suddenly get inspired to write a poem after reading a book and looking at some random photos. See section 3 of "The Digital Synaptic Neural Substrate: A New Approach to Computational Creativity" for more details.
Going back to John Roycroft’s problem, we can see that it used nine pieces in total. This rules out the possibility of Chesthetica having used an endgame tablebase in its composing process (which it never does) as they are currently, at best, limited to just seven pieces. This particular nine-piece selection limit was easily input into Chesthetica’s interface and the program was left to run on a single desktop computer (Intel(R) Core(TM) i5-4570 CPU @ 3.20GHz, Windows 10 Enterprise, 64-bit). There are theoretically about 5x1015 different ways these pieces could be configured on the board, by the way; so a purely random computational approach is futile in composing something legal and meaningful. Figure 2 shows how the program was set up via its interface.
Figure 2: Chesthetica v10.61 composing original studies using prespecified pieces
Within 24 hours of processing time, Chesthetica (v10.61) had produced dozens of studies using those pieces with White to play and win. Most were fairly interesting, at least to me, but the following few (see Figure 3) collectively, I think, would appeal to a wide selection or spectrum of chess players and maybe even some composers. Try to find the best move that wins. Some analysis is provided at the end of the article.
A selection of the original study-like constructs by Chesthetica, using the specific nine-piece set
If you found these challenging in any way or would have found them impressive if say, a child had come up with them instead of Chesthetica, then it only further illustrates the capabilities of the DSNS approach in the field of computational creativity (at least in chess). Also, bear in mind that there is presently no other computer program in the world that can do this. Even with very modest research backing and computing ability, Chesthetica has been producing thousands of original chess problems over the years with no limit in sight.
The DSNS approach is still very new in computational creativity and even more so within the larger and more generalized artificial intelligence (AI) field. Deep learning is now showing real potential thanks in part to improved hardware and massive amounts of training data but it is sobering to remember that it has its roots dating back to the 1940s. I, therefore, don’t expect the DSNS to even register on any corporation’s radar for another 20 or 30 years, at least, if at all. From an academic perspective, there is no value in ‘refining’ or ‘specializing’ Chesthetica further to compose master-level compositions as these products have little value to anyone but the handful of master composers and the relatively small subset of chess enthusiasts who have the patience to appreciate such problems and their intricate analyses. I also doubt any corporation with the funding necessary for such a specific project would see much value to it either.
The real value, therefore, lies in adapting the DSNS to other fields of interest where the automatic generation of creative objects could be useful and profitable. In principle, this can be anything ranging from antenna designs to protein structures. Having researched and built one such system for chess problems (i.e. Chesthetica), I can tell you that using it in more ‘serious’ domains would require quite a substantial investment of not only time and money but also expertise in programming and AI. Especially given the newer and more robust programming languages and platforms. It is unfortunate that most people barely understand (or even believe) that the DSNS works despite all Chesthetica has put out and are therefore unlikely to risk their time or money testing it further. My attempts to get funding for a ‘universal open-source DSNS engine’ have also failed repeatedly, and not unexpectedly so. This would have been software that people from any domain could use to generate the parameters necessary to help construct creative objects within their own domains.
For instance, a chair manufacturing company might be able to get some unique measurements, material types and colours it could use in its designs by combining say, photos of tables, classical music and paintings via the universal DSNS engine. A medical research team might be able to get some of the specifications of a drug it needs by using analogous input domains. Perhaps components of a bona fide cure for cancer are in some form in a piece of music or painting somewhere, staring right at us this whole time. Again, all of this requires a leap of faith and a lot more research and testing. Hence the decades between new ideas and their fruition. Imagine also what could perhaps be accomplished by combining the DSNS approach with advanced machine learning. Extremely large domain search spaces may not be an issue anymore. Once again, a lot more funding, research and testing are required for anything to possibly materialize. Regardless, I hope in 50 years ChessBase will still be around in some form and perhaps some 12-year-old child reading this now will remember to look up Chesthetica, either in amusement or in awe.
Sample images from DeviantArt.com: